Recent Developments in Inference: Practicalities for Applied Economics
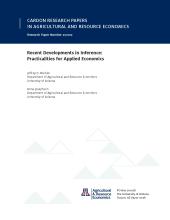
We provide a review of recent developments in the calculation of standard errors and test statistics for statistical inference. While much of the focus of the last two decades in economics has been on generating unbiased coefficients, recent years has seen a variety of advancements in correcting for non-standard standard errors. We synthesize these recent advances in addressing challenges to conventional inference, like heteroskedasticity, clustering, serial correlation, and testing multiple hypotheses. We also discuss recent advancements in numerical methods, such as the bootstrap, wild bootstrap, and randomization inference. We make three specific recommendations. First, applied economists need to clearly articulate the challenges to statistical inference that are present in data as well as the source of those challenges. Second, modern computing power and statistical software means that applied economists have no excuse for not correctly calculating their standard errors and test statistics. Third, because complicated sampling strategies and research designs make it difficult to work out the correct formula for standard errors and test statistics, we believe that in the applied economics profession it
should become standard practice to rely on asymptotic refinements to the distribution of an estimator or test statistic via bootstrapping. Throughout, we reference built-in and user-written Stata commands that allow one to quickly calculate accurate standard errors and relevant test statistics.
